8 Best Examples Of Data Science In Finance
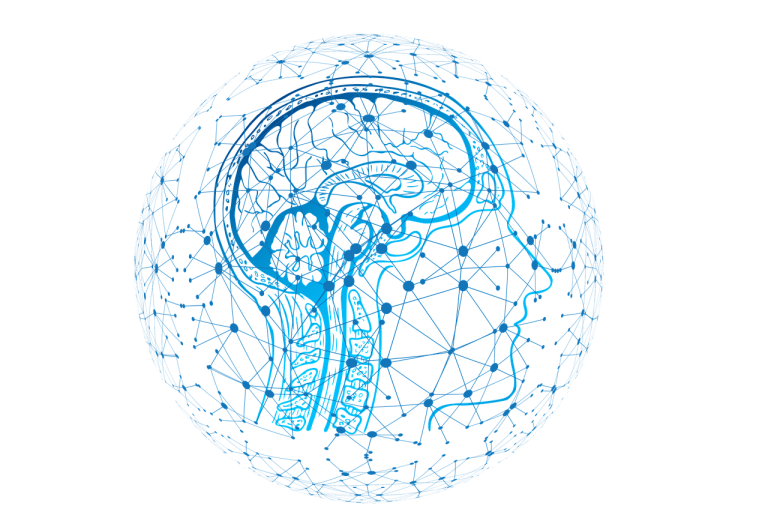
Data science in finance is aimed at extracting knowledge from a huge amount of data by employing mathematics and statistics. And many different techniques are employed to achieve this goal as good research leads to better outcomes leading to profit for financial institutions.
Data science has become extremely relevant in the finance sector, which is mainly used for Risk Management & Risk Analysis. Companies also evaluate data patterns through business intelligence software.
Through the use of Data Science, accuracy in identifying irregularities and fraud has increased. This has enabled reduced risks & scams, mitigating the losses, and preserving the financial institution’s image.
Data science and Finance go hand in hand as Finance is the hub of data. Financial institutions were among the earliest pioneers and users of data analytics.
In this blog, we’ll discuss the new applications of data science in the finance sector and how its developments in it revolutionize finance.
We’ll also discuss how various companies use data science to control their financial expenses.
What is Data Science?
According to Wikipedia-
Data science is an interdisciplinary field that uses scientific methods, processes, algorithms, and systems to extract knowledge and insights from many structural and unstructured data. Data science is related to data mining, machine learning and big data.
In simple words, Data science is the collection of data obtained from structured and unstructured sources so that valuable information is extracted. Data sources may include online or manual surveys, retail customer data, and social media usage information and actions.
These data are used for modelling actions of a network or client base to forecast future behaviour and patterns. This research may be extended to a specific sample community, such as retail clients or consumers of social media, weather forecasts, machine learning, and a vast array of other disciplines.
Such disciplines include ML, AI, statistics, mathematics, computer science and analytics, and many more.
What is the Role of Data Science in Finance?
Machine learning, big data, and artificial intelligence are fascinating, futuristic options for many developers, business people, and corporate employees.
Nonetheless, financial sector organizations, due to their security issues, often have resistance to newer technologies. The financial world, in fact, is mostly driven by cutting-edge developments.
While machine learning can render loan procedures efficient by reducing fraud, AI-powered applications can provide users with enhanced recommendations.
Since its inception, Data science has helped the evolution of many sectors. It is actually a thing that financial analysts have depended on data to draw many useful insights.
The growth of data science and machine learning has, however, brought about a huge enhancement in the industry. Today, automated algorithms and advanced analytical methods are being used together to get ahead of the competition more than ever.
To catch up with the pace of the latest trends and understand their usage, we’ll be discussing the value of data science in finance while giving many examples.
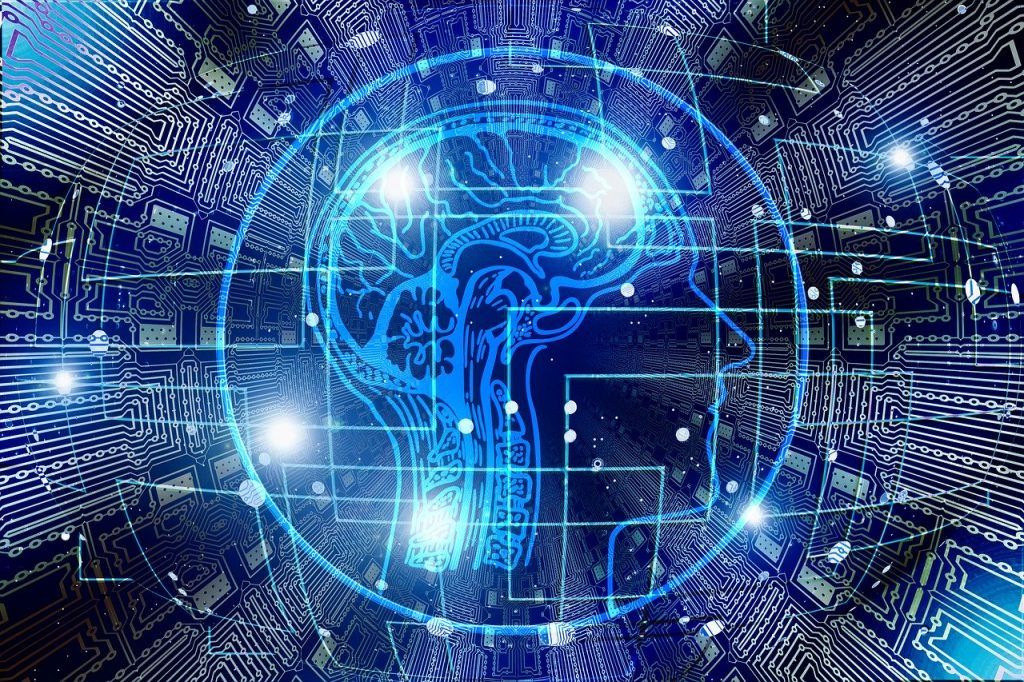
Usage of Data Science in Finance
Data Science is widely used in areas like risk analytics, customer management, fraud detection, and algorithmic trading. We will explore each of these and give you applications of Data Science in the finance Industry.
Risk Analytics
Initially, data were processed in batches and not in real-time, creating problems for different industries that needed data in real-time to obtain perspective into the current conditions.
Nevertheless, with improvements in technology and the growth of dynamic data pipelines, access to the data can now be made with minimal latency.
With this data science in finance applications, organizations are able to monitor purchases, credit scores, and other financial parameters without any latency issues.
Customer Analytics
Financial firms may make assumptions about how each customer is likely to behave based on previous behavioural patterns. Using socio-economic apps, they can break customers into clusters and make predictions of how much money each client expects to receive in the future.
The business separates customers into distinct classes based on certain attributes, such as age, employment, address, etc., using unsupervised Data science and machine learning techniques.
Then, by creating predictive models, they decide which of those characteristics is most important for each party. We allocate every client’s expected value according to this information.
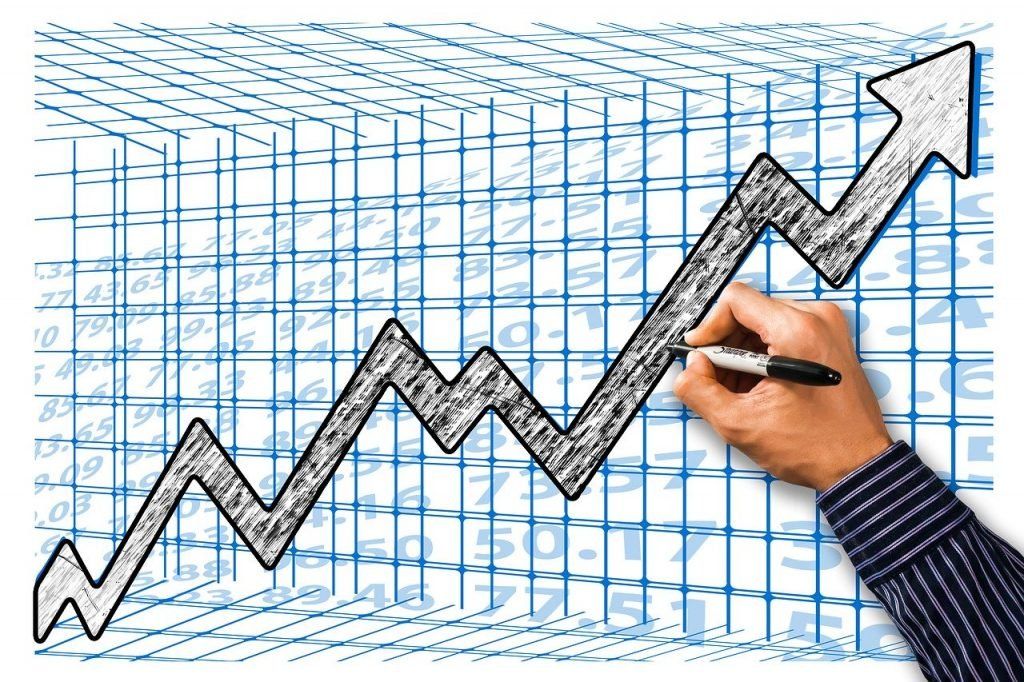
Fraud Detection
For finance firms, fraud is a major problem. Fraud risks have been growing with the number of transactions taking place. That being said, with the rise of big data and analytical software, financial firms have enabled themselves to keep a track of fraud.
Credit card fraud is among the most commonly practised frauds at financial firms. The identification of this form of fraud is attributed to developments in algorithms that have improved anomaly detection accuracies.
In addition, these detections warn the businesses about financial purchase irregularities, causing them to restrict the account to reduce the damages.
Algorithmic Trading
The most critical aspect of the financial markets is algorithmic trading. There are complex mathematical equations and lightning-speed computational methods in algorithmic trading which allow financial firms to formulate new trading techniques.
Big Data and Data science have had a major influence on algorithmic trading and it has become the most significant aspect of data science. The data present in algorithmic trading consists of a large number of data streams and includes a model that analyses the underlying streams of data and defines them.
The analytical engine’s goal is to make forecasts for the company’s future with a greater knowledge of large datasets.
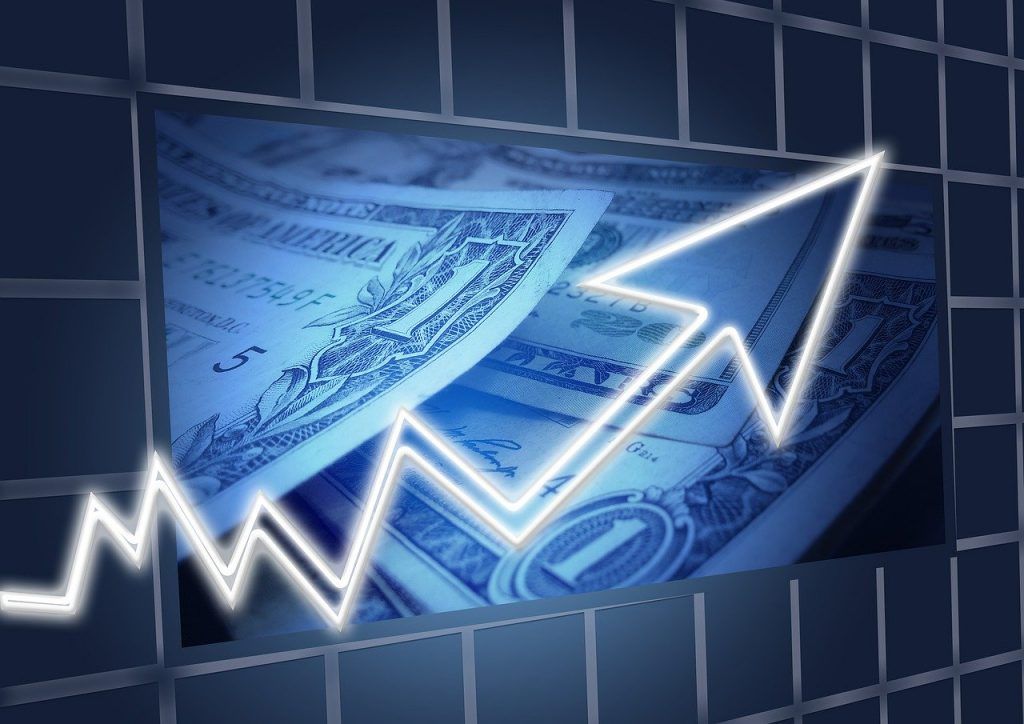
Deep Personalization and Customization
Companies recognize that one of the essential components to be sustainable in today’s economy is to increase interaction with their customers through high-quality, personal connections.
The aim is to examine the experience of digital clients and to adjust it taking into consideration the needs and desires of clients. AI is making major strides in understanding human language and emotion, which offers up an entirely new level of customer personalization.
Data engineers may also create models that analyze the actions of consumers and discover circumstances in which customers require financial advice.
Customer Data Management
There is a large amount of variation in the structure and volume of financial data today: from social media behaviour and smartphone interactions to business statistics and transaction information.
Finance professionals also have to deal with semi-structured or unstructured data and the retrieval of it manually is a significant obstacle.
For most organizations, however, it is clear that incorporating machine learning methods for process management is simply a requirement for extracting real data intelligence.
AI tools like Natural Language Processing, Data Mining, and Text Analytics, help turn data into information that leads to improved data governance and effective business solutions.
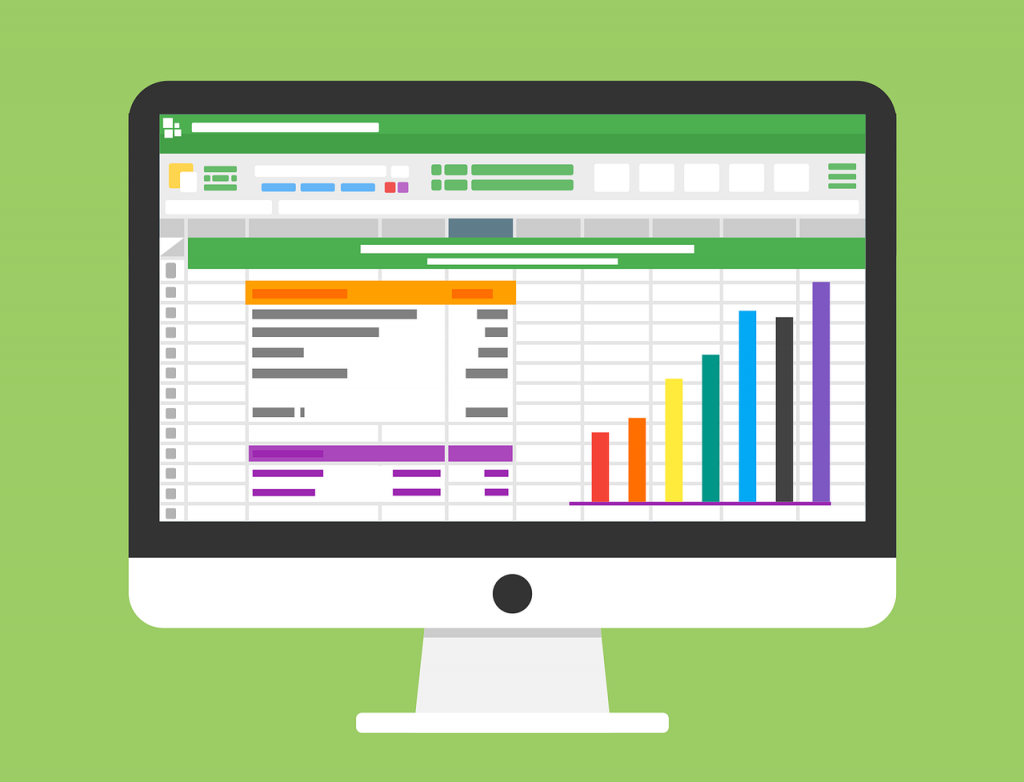
Predictive Analytics
Analytics has been at the heart of financial services today. Specific emphasis is required to be paid to predictive analytics which shows trends in the data that predict the future event that may occur now.
Through analyzing social media, news reports, and other information sources, these advanced analytics conquered core applications such as forecasting costs and lifetime value for consumers, potential life events, predicted turnover and stock market movements.
Most significantly, these approaches can help to answer the tough question-how to properly intervene.
Decision Making
Finance traders, executives, and stakeholders are required to judge market conditions on a daily basis and to make strategic decisions. This decision-making is only becoming intelligent with data science.
Propelled by past and present data, data science enables finance enthusiasts to determine at a given time the viability of trading.
Furthermore, these future predictions make it easy to judge moving markets like moving into a bear or bull market. Knowing these variables helps financial managers to build and invest in a viable portfolio of SIPs at the right time.
Certain analytics platforms go so far as to instruct when and how to proceed towards the next phase, when to withdraw your funds, and when to put in money.
To Conclude
Data science in finance offers a tremendous chance for financial firms to stay ahead of the competition and rebuild their companies-
- There are large quantities of constantly evolving financial data that generate a need for incorporating machine learning and AI technologies in multiple business areas.
- There are a number of Data Science positions in the Finance field. Data Science is also used in the area of risk management and analysis.
- Companies are increasingly using Data Science customer portfolio management to evaluate data patterns through business intelligence software.
- Financial firms use data analysis to identify unusual transactions and insurance scams for fraud detection.
- Data Science is also used in algorithmic trading, where machine learning plays a significant role in making the company’s future forecasts.
We are always trying our best to share valuable, informative, and useful posts with our readers. And we welcome your feedback about any incorrect information, or if you want to share more information about data science in finance.
You can comment in the comment section below and we make sure to reply at the earliest!
FAQ
Q1. Is Data Science a stressful job?
Ans- To put it in a precise manner, Data analysis is a difficult task. Amongst all else, the colossal volume of work, deadline constraints, and job demand from multiple sources and levels of management make a data scientist's job stressful.
Q2. Does Data Science pay well?
Ans- Despite a recent influx of early-career professionals, the median starting salary for a data scientist remains high at $95,000. Mid-level data scientist salary. The median salary for a mid-level data scientist is $130,000. If this data scientist is also in a managerial role, the median salary rises to $195,000.
Q3. Does Data Science require coding?
Ans- You need to have knowledge of various programming languages, such as Python, Perl, C/C++, SQL, and Java, with Python being the most common coding language required in data science roles. These programming languages help data scientists organize unstructured data sets.