Installation and Running of InternVideo2.5 on Windows
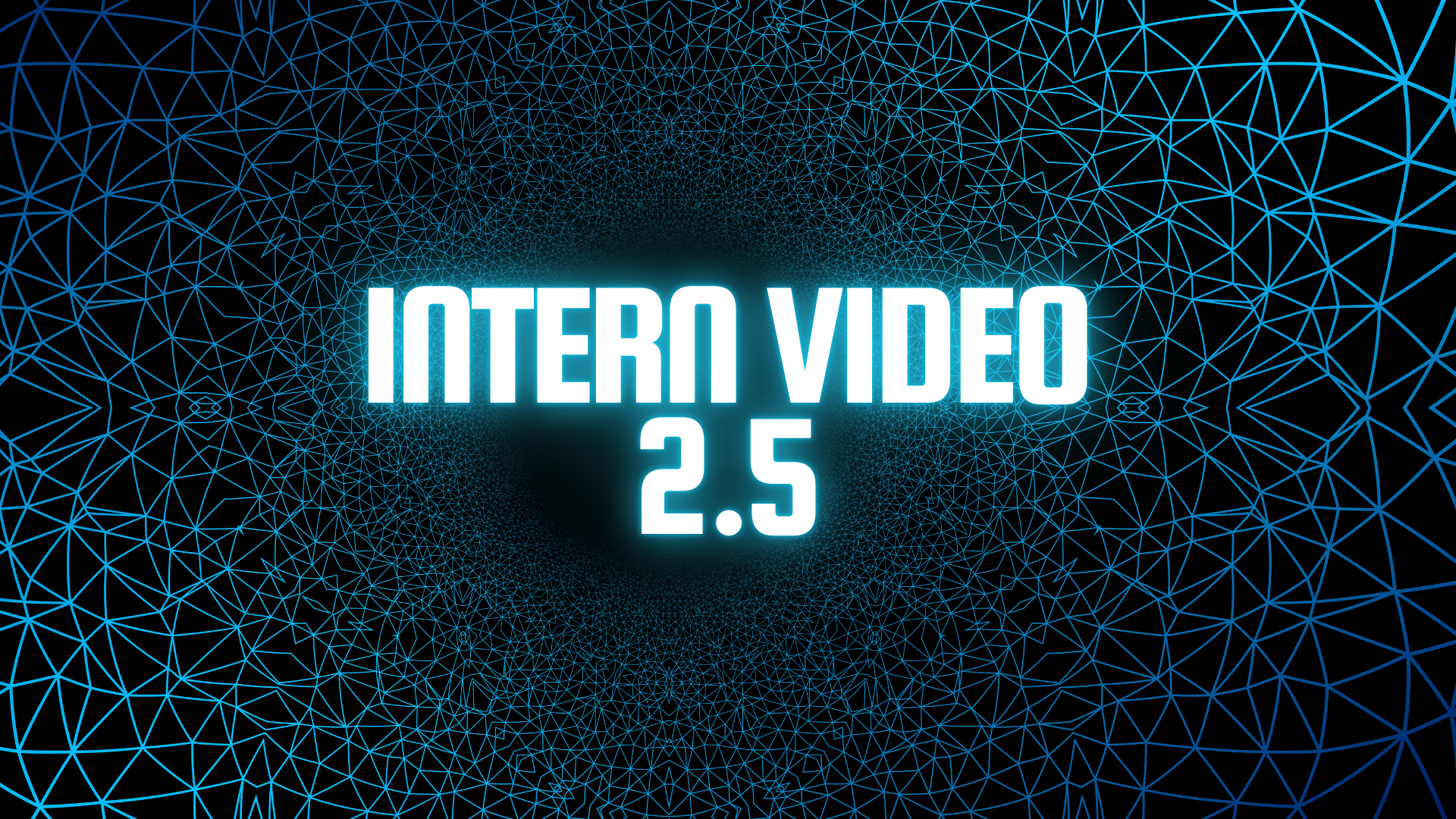
InternVideo2.5 represents an advanced video multimodal large language model (MLLM), extending upon InternVL2.5 with the incorporation of long and rich context (LRC) modeling.
This enhancement facilitates improved perception of fine-grained details and the comprehension of extended temporal structures.
What is InternVideo2.5?
InternVideo2.5 is an open-source video understanding model that excels at tasks like:
- Video classification
- Action recognition
- Temporal localization
- Video captioning
Built on PyTorch, it leverages advanced architectures like Vision Transformers (ViTs) and is pretrained on large datasets for robust performance.
Prerequisites
Before proceeding with the installation, confirm that your system satisfies the following requirements:
- Operating System: Windows 10 or later
- Python Version: 3.8 or newer
- CUDA: Version 11.0 or higher (for GPU acceleration)
- Storage Requirements: A minimum of 20GB available for the model and dependencies
- RAM: At least 16GB (recommended)
- GPU (Optional but Recommended): NVIDIA GPU with a minimum of 8GB VRAM
Step 1: Install Python and pip
If Python is not already installed, obtain the latest version from the official Python website. Ensure that the installation process includes adding Python to the system's PATH environment variable.
To verify installation, execute the following commands in a command prompt:
python --version
pip --version
Step 2: Establish a Virtual Environment
Creating a virtual environment is strongly recommended to encapsulate dependencies specific to InternVideo2.5 and mitigate compatibility issues.
cd your_project_directory
python -m venv internvideo_env
internvideo_env\Scripts\activate
Step 3: Install Required Dependencies
Utilize pip to install the essential packages:
pip install transformers==4.40.1 av imageio decord opencv-python flash-attn --no-build-isolation
Step 4: Model Acquisition
Retrieve the InternVideo2.5 model from the Hugging Face Model Hub:
from transformers import AutoModel, AutoTokenizer
model_path = 'OpenGVLab/InternVideo2_5_Chat_8B'
tokenizer = AutoTokenizer.from_pretrained(model_path, trust_remote_code=True)
model = AutoModel.from_pretrained(model_path, trust_remote_code=True).half().cuda()
Step 5: Environment Configuration
Ensure that system environment variables are correctly configured for CUDA:
setx PATH "%PATH%;C:\Program Files\NVIDIA GPU Computing Toolkit\CUDA\v11.0\bin"
Step 6: Data Preparation
Ensure input video files are in a supported format before processing with InternVideo2.5.
Step 7: Implementation Examples
Example 1: Extracting Key Frames from a Video
import cv2
import numpy as np
def extract_key_frames(video_path, output_folder, frame_interval=30):
cap = cv2.VideoCapture(video_path)
frame_count = 0
while cap.isOpened():
ret, frame = cap.read()
if not ret:
break
if frame_count % frame_interval == 0:
output_path = f"{output_folder}/frame_{frame_count}.jpg"
cv2.imwrite(output_path, frame)
frame_count += 1
cap.release()
extract_key_frames("sample_video.mp4", "frames_output")
Example 2: Speech Transcription via OpenAI Whisper
import whisper
model = whisper.load_model("base")
result = model.transcribe("sample_video.mp4")
print(result["text"])
Example 3: Automated Video Captioning with InternVideo2.5
import torch
from some_video_processing_module import load_video
def generate_video_captions(video_path):
pixel_values, num_patches_list = load_video(video_path, num_segments=128, max_num=1)
pixel_values = pixel_values.to(torch.bfloat16).to(model.device)
question = "Describe this video in detail."
video_prefix = "".join([f"Frame{i+1}: \n" for i in range(len(num_patches_list))])
question = video_prefix + question
output, _ = model.chat(tokenizer, pixel_values, question, generation_config, num_patches_list=num_patches_list, history=None, return_history=True)
print(output)
generate_video_captions("sample_video.mp4")
Step 8: Executing the Model
To execute InternVideo2.5, run the relevant script:
python your_script_name.py
Running Your First Video Analysis
Input Video Preparation
- Supported formats: MP4, MOV, AVI
- Resolution: 1920x1080 or lower recommended
- Duration: Optimized for 30s-5min clips
# Enhanced video loader with error handling
def safe_load_video(path):
try:
vr = VideoReader(path, ctx=cpu(0))
return vr
except Exception as e:
print(f"Error loading {path}: {str(e)}")
return None
Comprehensive Processing Pipeline
- Frame Extraction Strategies
- Fixed interval sampling
- Dynamic scene detection
- Keyframe extraction
- Multi-Modal Prompt Engineering
prompt_template = """
Analyze this video from {timestamp} to {duration}:
{query}
Consider these aspects:
- Object interactions
- Temporal relationships
- Scene context
- Action sequences
"""
Advanced Configuration Tips
Performance Optimization
Technique | Speed Gain | Quality Impact |
---|---|---|
Mixed Precision (FP16) | 2.1x | Minimal |
Flash Attention 2 | 1.8x | None |
Batch Processing | 3.5x | Context Loss |
# Enable advanced optimizations
model = AutoModel.from_pretrained(...).half().to('cuda')
model = torch.compile(model) # PyTorch 2.0 feature
Memory Management
- Gradient Checkpointing:
model.gradient_checkpointing_enable()
- Frame Chunking: Process video in 30s segments
- VRAM Monitoring: Use
nvidia-smi -l 1
Troubleshooting Common Issues
Error: "CUDA Out of Memory"
- Reduce batch size:
num_segments=64
- Enable garbage collection:
import gc
gc.collect()
torch.cuda.empty_cache()
Video Processing Errors
- Corrupted Files: Use
ffprobe your_video.mp4
Codec Issues: Convert to H.264 using FFmpeg:
ffmpeg -i input.avi -c:v libx264 output.mp4
Citation
If utilizing InternVideo2.5 for research purposes, please cite:
@article{wang2025internvideo,
title={InternVideo2.5: Empowering Video MLLMs with Long and Rich Context Modeling},
author={Wang, Yi and Li, Xinhao and Yan, Ziang and others},
journal={arXiv preprint arXiv:2501.12386},
year={2025}
}
Real-World Application
- Content Moderation: Automatically detect policy violations in video uploads
- Sports Analytics: Track player movements and game dynamics
- Educational Content: Generate automatic lecture summaries with key concepts
# Example: Educational Video Analyzer
def generate_lecture_summary(video_path):
analysis = model.analyze(video_path)
return f"""
Lecture Summary:
- Key Topics: {analysis['topics']}
- Visual Aids: {analysis['diagrams']}
- Recommended Study Points: {analysis['important_concepts']}
"""
Conclusion
By adhering to the aforementioned steps, users can successfully install and execute InternVideo2.5 on a Windows system, leveraging its capabilities for advanced video analysis and multimodal comprehension.