MCP vs API: Differences, Similarities, and Benefits
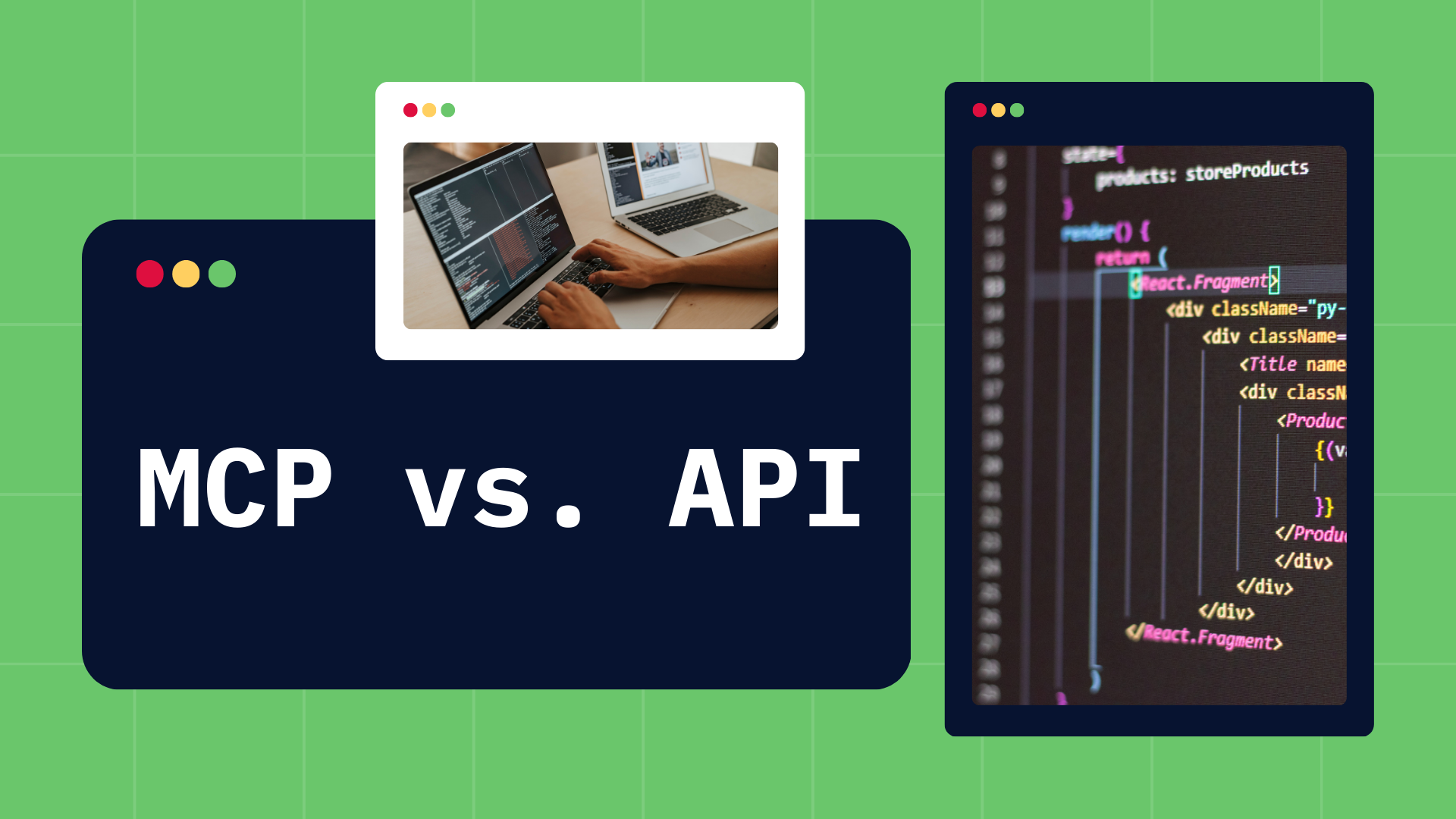
The landscape of software integration and automation is evolving rapidly, especially with the rise of AI-driven systems. Two key technologies at the center of this transformation are MCP (Model Context Protocol) and API (Application Programming Interface).
While both act as communication bridges between software systems, their design, use cases, and advantages vary significantly.
This guide offers a deep dive into MCP vs API — comparing their architectures, similarities, differences, and the unique benefits each brings to modern software development and AI integration.
Understanding the Basics
What is an API?
An API (Application Programming Interface) is a set of rules and protocols that allows software applications to communicate. APIs define how to request services or data, what data formats to use, and how responses are returned.
APIs are crucial in modern development, enabling applications to integrate third-party services without starting from scratch. They can be public (open), private (internal), or partner-specific. Common formats include REST, SOAP, and GraphQL.
What is MCP?
MCP (Model Context Protocol) is an open standard created to streamline AI integration. It acts as a universal translator, allowing AI models to interact with various tools and data sources using a standardized protocol—think of it as a “USB-C for AI integrations.”
Developed by Anthropic and open-sourced, MCP is tailored for environments where AI agents must dynamically interact with numerous tools. Rather than writing custom code for each tool, developers can use MCP to enable scalable AI-driven automation.
Architectural Overview
Aspect | API | MCP |
---|---|---|
Design | Software-to-software communication interface | Protocol for AI-to-software communication |
Primary User | Developers | AI agents and hosts |
Integration | Unique, per-API custom implementations | Unified protocol across tools |
Standardization | Varies (REST, SOAP, etc.) | Single open standard |
API Architecture
APIs follow a client-server model: the client (app) sends a request to the API server, which processes and responds. APIs expose specific endpoints for data or functionality and may use JSON, XML, or other formats.
MCP Architecture
MCP also follows a client-server model, but with AI-specific enhancements:
- MCP Server: Adapts applications to expose their capabilities in a standardized format, translating AI requests into tool-specific actions.
- MCP Client: Typically embedded within the AI agent, it communicates with MCP servers to send instructions and receive data.
- Protocol: Defines message formats, discovery rules, and communication channels (HTTP, WebSockets, etc.), usually structured with JSON Schema.
Key Differences Between MCP and API
A. Purpose and Target Audience
- API: Designed for developer use, often requires reading documentation and writing custom code.
- MCP: Designed for AI agents, abstracting tool-specific logic for scalable integration.
B. Integration Complexity
- API: Each API requires different authentication, data formats, and handling.
- MCP: Standardized protocol allows quick integration with minimal configuration.
C. Flexibility and Scalability
- API: Monolithic APIs are harder to scale and update.
- MCP: Based on microservices, enabling modular scalability and easier maintenance.
D. Protocol and Communication
- API: Uses REST, SOAP, GraphQL—typically synchronous and endpoint-based.
- MCP: Uses a unified, dynamic protocol supporting real-time discovery and updates.
E. Automation and AI Workflows
- API: Requires manual scripting for automation.
- MCP: Supports AI-driven, adaptive workflows without custom scripting.
F. Fault Tolerance
- API: Single-point failures can impact the entire system.
- MCP: Issues are isolated to individual services, improving resilience.
Similarities Between MCP and API
Despite different approaches, MCP and APIs share core concepts:
- Client-server architecture
- Encapsulation of complexity
- Support for security standards
- Documentation for integration
- Bridging between systems
Detailed Comparison Table
Feature | API | MCP |
---|---|---|
Primary User | Developers, applications | AI agents, hosts |
Integration | Custom code per API | Standardized across tools |
Protocol | REST, SOAP, GraphQL | Single open protocol using JSON Schema |
Discovery | Manual via documentation | Dynamic via AI queries |
Scalability | Limited by monolithic architecture | Microservice-friendly |
Flexibility | Rigid; changes can affect entire system | Modular and easily upgradable |
Automation | Manual scripting | AI-driven dynamic chaining |
Fault Isolation | Low | High |
Maturity | High | Emerging |
Best Use Case | Simple, stable integrations | Complex, AI-powered environments |
Benefits of Each Approach
Benefits of APIs
- Proven Technology: Mature and reliable.
- Developer Control: Allows fine-tuned customization.
- Security: Established standards and frameworks.
- Ecosystem: Abundant resources and community support.
Benefits of MCP
- AI-First Design: Seamless for AI agents and hosts.
- Standardization: One protocol for all tools.
- Dynamic Discovery: Real-time exploration of available functions.
- Scalability: Modular and easy to extend.
- Ideal for Automation: Native support for chaining complex tasks.
Practical Use Cases
When to Use an API
- Integrating a single tool like a payment or email service.
- Building tightly controlled workflows.
- Needing well-documented, stable tools.
- Performing static integrations that won’t change often.
When to Use MCP
- Enabling AI assistants to interact with multiple tools.
- Creating adaptive workflows that evolve.
- Scaling integrations quickly without new code.
- Orchestrating actions across tools using AI logic.
Hybrid Approaches
MCP often works on top of existing APIs. An MCP server might wrap an app’s API, making it AI-friendly. APIs provide raw access, and MCP enables AI to use that access intelligently and dynamically.
Limitations and Considerations
APIs
- Custom Effort: New APIs require new code.
- Inconsistencies: Each API has its own style.
- Not AI-Optimized: Manual setup limits adaptability.
MCP
- New Standard: Still maturing and not yet widely adopted.
- Adoption Curve: Requires providers to implement MCP servers.
- Overhead for Simple Tasks: May be unnecessary for basic integrations.
The Future: AI-First Integration
As AI continues to drive automation, there’s a growing shift from developer-centric integration (APIs) to AI-centric protocols like MCP. This transition supports scalable, real-time, and adaptive workflows essential for next-gen intelligent systems.
MCP is a forward-looking protocol built for this reality—allowing AI agents to go beyond static data exchange and take meaningful actions across tools. That said, APIs will continue to serve as the backbone of functionality that MCP builds upon.
Conclusion
APIs and MCP are complementary pillars of software integration:
- APIs offer stable, flexible integrations for developer-driven environments.
- MCP powers scalable, AI-driven automation in dynamic ecosystems.
Use APIs for traditional integrations where control, maturity, and customization matter.
Use MCP to enable AI agents to act autonomously across systems, unlocking true automation at scale.