MCP vs API: Why They Are Not the Same?
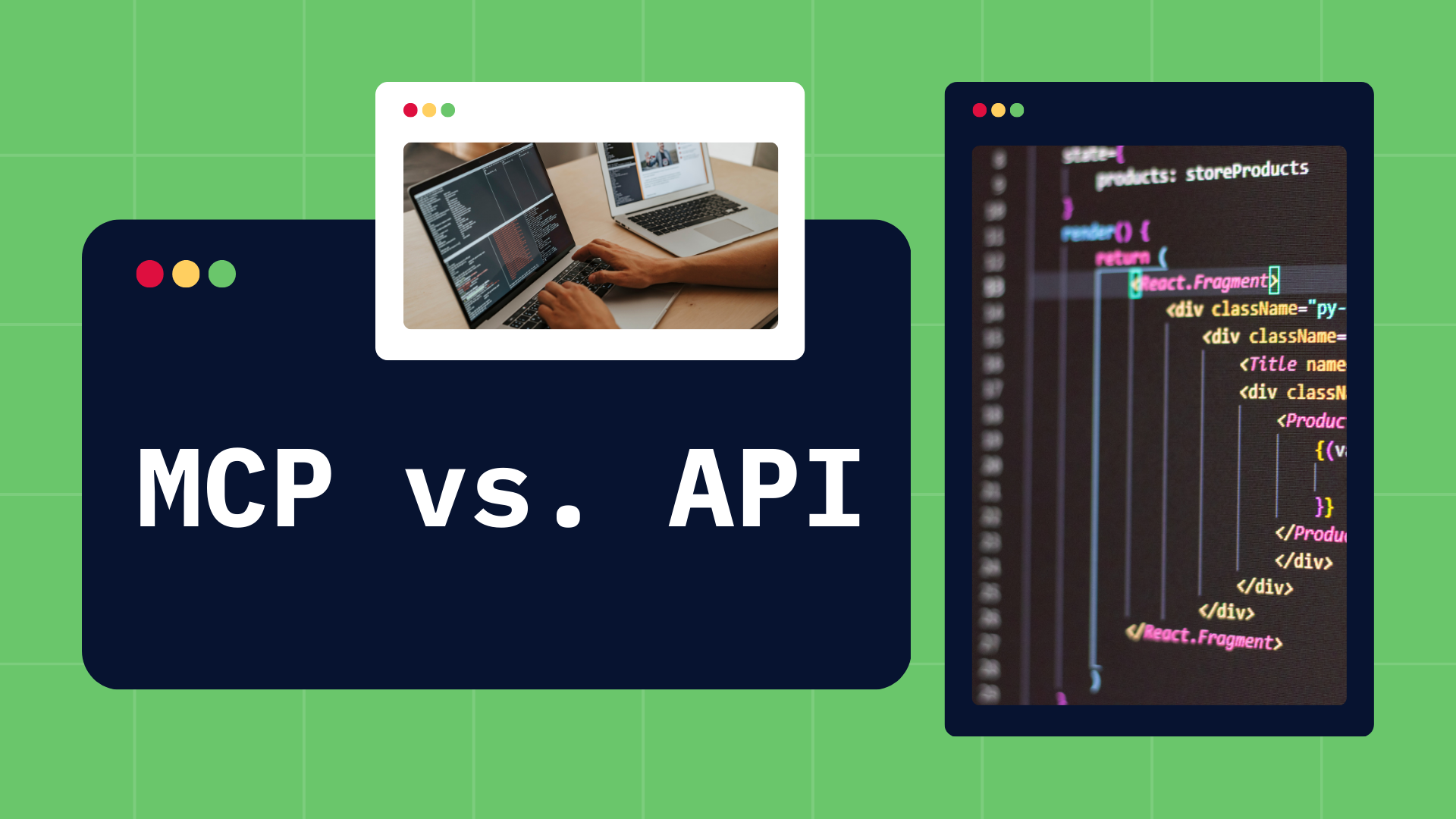
The rapid evolution of software integration and artificial intelligence (AI) has introduced new paradigms for how systems communicate and collaborate. Two terms that frequently arise in this context are API (Application Programming Interface) and MCP (Model Context Protocol).
While both facilitate connections between software components, they serve fundamentally different purposes, are designed for different users, and operate at distinct layers of abstraction.
This article explores the differences between MCP and API, their design philosophies, technical structures, and practical use cases—clarifying why they are not interchangeable.
Understanding the Basics
What is an API?
An API (Application Programming Interface) is a set of definitions and protocols that enables two software components to communicate. APIs structure requests and responses so that applications can exchange data and trigger actions without knowing each other's internal logic.
APIs typically follow a client-server model:
- Client: The application making the request.
- Server: The application responding to the request.
Types of APIs:
- REST APIs – Use HTTP, focus on resources, and are stateless.
- SOAP APIs – Use XML messaging, often more rigid.
- RPC APIs – Invoke functions on remote systems.
- WebSocket APIs – Enable two-way communication for real-time systems.
What is MCP?
MCP (Model Context Protocol), developed by Anthropic, is an open standard designed for AI models to interact with external systems—like APIs, databases, or tools—without needing custom integration code. MCP abstracts underlying technical details and enables AI to perform tasks via a unified, intention-based interface.
MCP consists of:
- Host – The AI environment (e.g., Claude).
- MCP Client – Embedded within the AI model to structure requests.
- MCP Server – Connects the model with external systems.
MCP primitives include:
- Clients – Roots (secure file access), Sampling (task guidance).
- Servers – Prompts (instructions), Resources (data objects), Tools (functions the AI can call).
Key Differences Between MCP and API
1. Purpose and Design Philosophy
Aspect | API | MCP |
---|---|---|
Primary User | Developers | AI models and agents |
Goal | Enable software communication | Enable AI-driven autonomy |
Abstraction | Low-level, technical | High-level, intention-based |
- APIs are developer-oriented and focus on manual, structured integration.
- MCP is designed for AI, emphasizing intuitive tool use based on intent rather than syntax.
2. Integration and Flexibility
- API integration involves unique authentication, data formats, and code for every service.
- MCP allows standardized, scalable AI access to tools via one protocol—dramatically simplifying integration efforts.
3. Protocols and Architecture
Aspect | Traditional API | MCP (Modern Protocol) |
---|---|---|
Architecture | Monolithic/single service | Microservices/modular |
Scalability | Whole system | Individual services |
Protocols | SOAP, REST, RPC, WebSocket | REST, GraphQL, intention-based |
Management | Manual | Automated via gateways |
Deployment | Full app redeploy | Modular updates |
Fault Isolation | Broad impact | Isolated failures |
MCP reflects modern architecture needs—resilient, loosely coupled, and scalable.
4. Usability for AI
- APIs are not inherently AI-friendly; AI must be explicitly trained to interact with them.
- MCP provides AI with discoverable, dynamic access to tools using standardized semantics.
5. Use Cases
Scenario | API Approach | MCP Approach |
---|---|---|
SaaS integrations | Custom code per API | Standard protocol, no custom code needed |
Multi-step workflows | Manually scripted | AI chains actions dynamically |
One-off simple tasks | Direct call via API | MCP if infrastructure is in place |
Adapting to new services | Manual code for each | AI can use new tool immediately via MCP |
How MCP and API Work Together?
MCP does not replace APIs—it leverages them. In most use cases, MCP servers interact with traditional APIs under the hood, handling authentication, parsing, and error management. This makes AI integration much smoother.
“MCP is like a specialized vehicle AI uses to drive on data highways (APIs). It makes navigating complex systems safe and intuitive for the AI.”
Technical Comparison: MCP vs API
API: Key Characteristics
- Endpoints: URL-based, operation-specific.
- Authentication: API keys, OAuth, etc.
- Documentation: Manual reading and implementation required.
- Error Handling: Must be explicitly coded.
- Data Format: JSON, XML—strict schemas.
MCP: Key Characteristics
- Tool Discovery: AI can dynamically detect capabilities.
- Intent-Based: High-level commands over granular parameters.
- Standardization: Uniform protocol across services.
- Security: Managed via gateways and policy layers.
Practical Scenarios
Scenario 1: Integrating Multiple SaaS Tools
- API: Developer effort scales linearly with each tool.
- MCP: Once MCP servers are in place, AI can access all tools immediately.
Scenario 2: Multi-Step Workflows
- API: Predefined scripts and error handling required.
- MCP: AI creates workflows in real time using tool chaining.
Scenario 3: Adapting to New Services
- API: Code, debug, and maintain each new integration.
- MCP: Just add an MCP server—AI adapts instantly.
MCP in the Context of Modern Software Architecture
MCP aligns with trends in cloud-native and microservices architecture:
- Resilience: Isolates faults to specific tools/services.
- Scalability: Services scale independently.
- Automation: Tool discovery and usage are AI-driven.
In contrast, traditional APIs struggle with dynamic scaling and integration without substantial developer involvement.
Why MCP and API Are Not the Same?
1. Layers of Abstraction
- API: Detailed, operation-specific instructions.
- MCP: High-level goals and actions.
2. Target User
- API: Built for human developers.
- MCP: Built for AI agents and models.
3. Integration Strategy
- API: Custom integration each time.
- MCP: Dynamic, AI-driven tool discovery and usage.
4. Future-Proofing
MCP absorbs inconsistencies between systems, making it more adaptable to fast-evolving tech stacks.
Common Misconceptions
“Is MCP just another API?”
No—MCP is a protocol that may utilize APIs internally but offers a different abstraction layer focused on AI autonomy.
“Can MCP work without APIs?”
Unlikely. MCP still depends on APIs for data and actions; it simply wraps them in a format accessible to AI.
Summary Table: MCP vs API
Feature | API | MCP |
---|---|---|
Primary User | Developer | AI model/agent |
Integration Effort | High | Low, reusable interface |
Abstraction Level | Low (technical) | High (intent-based) |
Flexibility | Rigid | Adaptive |
Scalability | Whole system | Individual services |
Fault Tolerance | Broad impact | Isolated |
Use Case | General software communication | Autonomous AI tool usage |
Example | REST API for Slack | MCP server for Slack used by an AI agent |
Conclusion
MCP and APIs are both foundational to modern digital ecosystems—but they are not the same.
- APIs are designed for developers, exposing technical operations for software-to-software interaction.
- MCP is designed for AI models, abstracting complexity and enabling intuitive, autonomous tool usage.
As AI takes a more central role in automation, the need for frameworks like MCP becomes more pressing. MCP doesn’t replace APIs—it enhances them, allowing AI to unlock their power without requiring manual effort for each integration.
Understanding the distinction between MCP and API is essential for building scalable, resilient, and intelligent systems that keep pace with rapid technological change.