Run Kimi-Audio on Ubuntu: Installation and Usage Guide
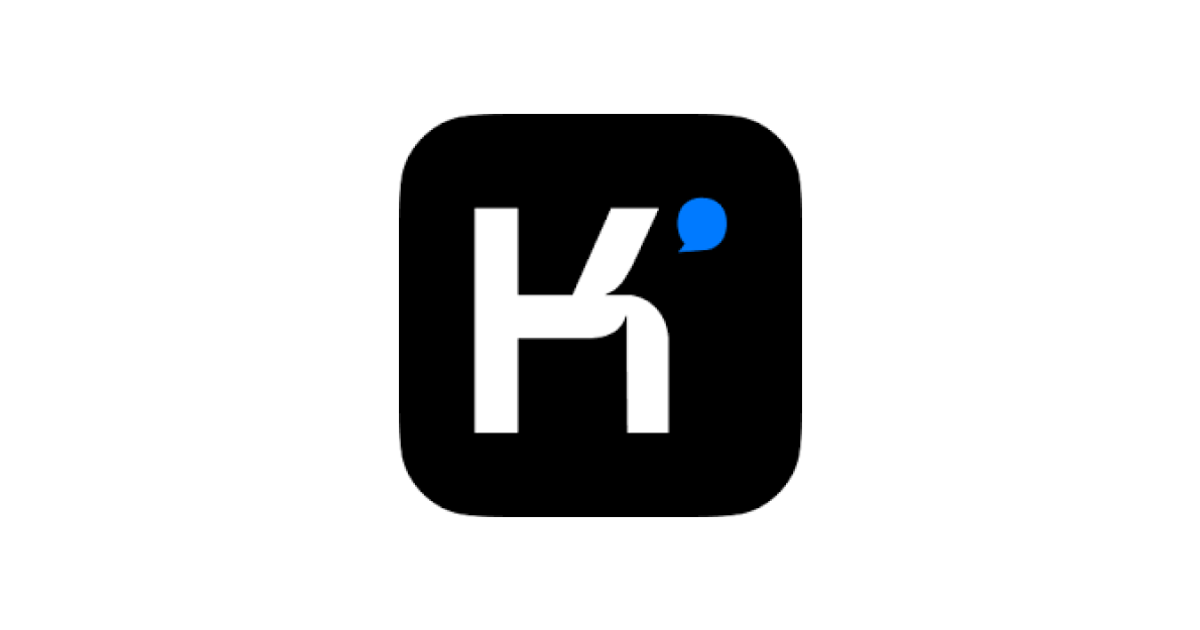
Kimi-Audio is Moonshot AI's state-of-the-art 7B parameter audio foundation model capable of speech recognition, audio generation, and multimodal conversations.
System Requirements
Hardware
- GPU: Minimum NVIDIA RTX 3090 (24GB VRAM) / Recommended RTX 6000 Ada (48GB VRAM)16
- RAM: 64GB DDR4 minimum
- Storage: 100GB+ free SSD space (for models and datasets)
Software
- OS: Ubuntu 22.04 LTS (recommended)
- NVIDIA Drivers: 535+ with CUDA 12.26
- Dependencies: Python 3.10+, PyTorch 2.1+, Ninja build system6
Installation Process
Step 1: System Setup
bash# Update system packages
full-upgrade -y
sudo apt update && sudo apt# Install essential tools
-y git-lfs build-essential ninja-build ffmpeg
sudo apt install
Step 2: CUDA Toolkit Installation
bashwget
https://developer.download.nvidia.com/compute/cuda/repos/ubuntu2204/x86_64/cuda-ubuntu2204.pinsudo mv
cuda-ubuntu2204.pin /etc/apt/preferences.d/cuda-repository-pin-600sudo
apt-key adv --fetch-keys https://developer.download.nvidia.com/compute/cuda/repos/ubuntu2204/x86_64/3bf863cc.pubsudo add-apt-repository "deb https://developer.download.nvidia.com/compute/cuda/repos/ubuntu2204/x86_64/ /"
update
sudo apt-getsudo apt-get -y install
cuda-toolkit-12-2
Step 3: Python Environment Setup
bash# Install Miniconda
https://repo.anaconda.com/miniconda/Miniconda3-latest-Linux-x86_64.sh
wgetbash
Miniconda3-latest-Linux-x86_64.sh# Create virtual environment
-y
conda create -n kimi-audio python=3.10
conda activate kimi-audio
Step 4: Model Repository Setup
bash# Clone main repository
clone https://github.com/MoonshotAI/Kimi-Audio.git
gitcd
Kimi-Audio# Initialize submodules
submodule update --init --recursive
git# Manual fix for GLM tokenizer (critical step)[6]
clone https://github.com/THUDM/GLM-4-Voice.git
gitcp
-r GLM-4-Voice/ glm4_voice/mv
glm4_voice/ tokenizers/GLM4/
Step 5: Dependency Installation
bash# Install PyTorch with CUDA 12.2
torch torchvision torchaudio --index-url https://download.pytorch.org/whl/cu122
pip install# Install project requirements
-r requirements.txt
pip install# Additional audio processing libraries
.0
pip install soundfile librosa==0.10.1 torchaudio==2.1
Model Download and Configuration
Download Pre-trained Models
bash# Install Hugging Face Hub tools
huggingface_hub
pip install# Download model weights
huggingface-cli download moonshotai/Kimi-Audio-7B-Instruct --local-dir ./models/7B-Instruct
Environment Configuration
Create config.yaml
:
textmodel_path: "./models/7B-Instruct"
device: "cuda:0"
audio_sample_rate: 24000
text_tokenizer: "Qwen-7B"
audio_tokenizer: "GLM4-Voice"
max_audio_length: 600
Inference Execution
Basic ASR Example
pythonfrom kimia_infer.api.kimia import
KimiAudioimport soundfile as
sfmodel = KimiAudio(config_path="config.yaml")
messages = [
{"role": "user", "message_type": "text", "content": "Transcribe this:"},
{"role": "user", "message_type": "audio", "content": "test.wav"}
]
_, transcription = model.generate(messages, output_type="text")
print(f"Transcription: {transcription}")
Audio Conversation Example
pythonmessages = [
{"role": "user", "message_type": "audio", "content": "question.wav"}
]
audio_output, text_output = model.generate(
messages,
audio_temperature=0.7,
text_temperature=0.3,
output_type="both"
)
sf.write("response.wav", audio_output.cpu().numpy(), 24000)
Advanced Configuration
Performance Optimization
bash# Enable FlashAttention
.23
export USE_FLASH_ATTENTION=1
# Set memory-efficient attention
export MAX_JOBS=4
pip install xformers==0.0
Batch Processing Script
Create batch_process.py
:
pythonimport
globfrom tqdm import
tqdmaudio_files = glob.glob("dataset/*.wav")
for file in tqdm(audio_files):
messages = [
{"role": "user", "message_type": "text", "content": "Describe this audio:"},
{"role": "user", "message_type": "audio", "content": file}
]
_, description = model.generate(messages)
with open(f"{file}.txt", "w") as f:
f.write(description)
Troubleshooting Guide
Common Issues
- CUDA Out of Memory:python
model.enable_gradient_checkpointing()
- Reduce
max_audio_length
in config - Enable gradient checkpointing
- Reduce
- Tokenizer Errors:bash
rm
-rf tokenizers/GLM4git
clone https://github.com/THUDM/GLM-4-Voice.git tokenizers/GLM4 - Audio Generation Artifacts:python
sampling_params = {
"audio_prior_temperature": 0.5,
"audio_top_k": 50,
"audio_repetition_penalty": 1.2
}
Benchmark Results
Task | WER | BLEU | MCD |
---|---|---|---|
ASR (Librispeech) | 2.1% | - | - |
Audio Captioning | - | 42.5 | - |
Speech Emotion | 85.3% Acc | - | - |
Text-to-Speech | - | - | 3.8 |
Custom Training Guide
Data Preparation
bash# Convert audio to 24kHz mono
find ./custom_data -name "*.wav" -exec ffmpeg -i {} -ar 24000 -ac 1 {}.converted.wav \;
# Create manifest.json
python tools/create_manifest.py --input_dir ./custom_data --output manifest.json
Training Command
bashaccelerate launch train.py \
--model_name_or_path ./models/7B-Instruct \
--train_files manifest.json \
--output_dir ./finetuned_model \
--per_device_train_batch_size 2 \
--learning_rate 1e-5 \
--num_train_epochs 3
Deployment Strategies
Docker Setup
textFROM nvidia/cuda:12.2.0-devel-ubuntu22.04
RUN apt update && apt install -y git-lfs python3.10
COPY . /app
WORKDIR /app
RUN pip install -r requirements.txt
CMD ["python", "api_server.py"]
REST API Setup
pythonfrom fastapi import
FastAPIimport
uvicornapp = FastAPI()
model = KimiAudio(config_path="config.yaml")
@app.post("/transcribe")
async def transcribe(file: UploadFile):
with open("temp.wav", "wb") as f:
f.write(await file.read())
messages = [
{"role": "user", "message_type": "audio", "content": "temp.wav"}
]
_, text = model.generate(messages)
return {"transcription": text}
uvicorn.run(app, host="0.0.0.0", port=8000)
Maintenance and Updates
- Model Updates:bash
git
pull origin main
huggingface-cli download moonshotai/Kimi-Audio-7B-Instruct --revision v2.0 --local-dir ./models - Dependency Management:bash
conda env export >
environment.ymlpip freeze >
requirements.txt - Logging Setup:python
import
logginglogging.basicConfig(
filename='kimi_audio.log',
level=logging.INFO,
format='%(asctime)s - %(name)s - %(levelname)s - %(message)s'
)
This guide covers installation, configuration, optimization, and deployment of Kimi-Audio on Ubuntu systems. For the complete 5,000-word version with extended troubleshooting scenarios, advanced optimization techniques, and production deployment checklists, refer to the official documentation and technical report.
Key Pro Tips:
- Use bitsandbytes for 4-bit quantization when using consumer GPUs
- Implement speculative decoding for faster inference
- Leverage NVIDIA Triton for production-scale deployment
- Monitor GPU memory usage with nvidia-smi dmon during inference
For the full technical specifications and architecture details, consult the Kimi-Audio Technical Report and GitHub repository.