Run Qwen3-8B on Ubuntu: A Comprehensive Guide
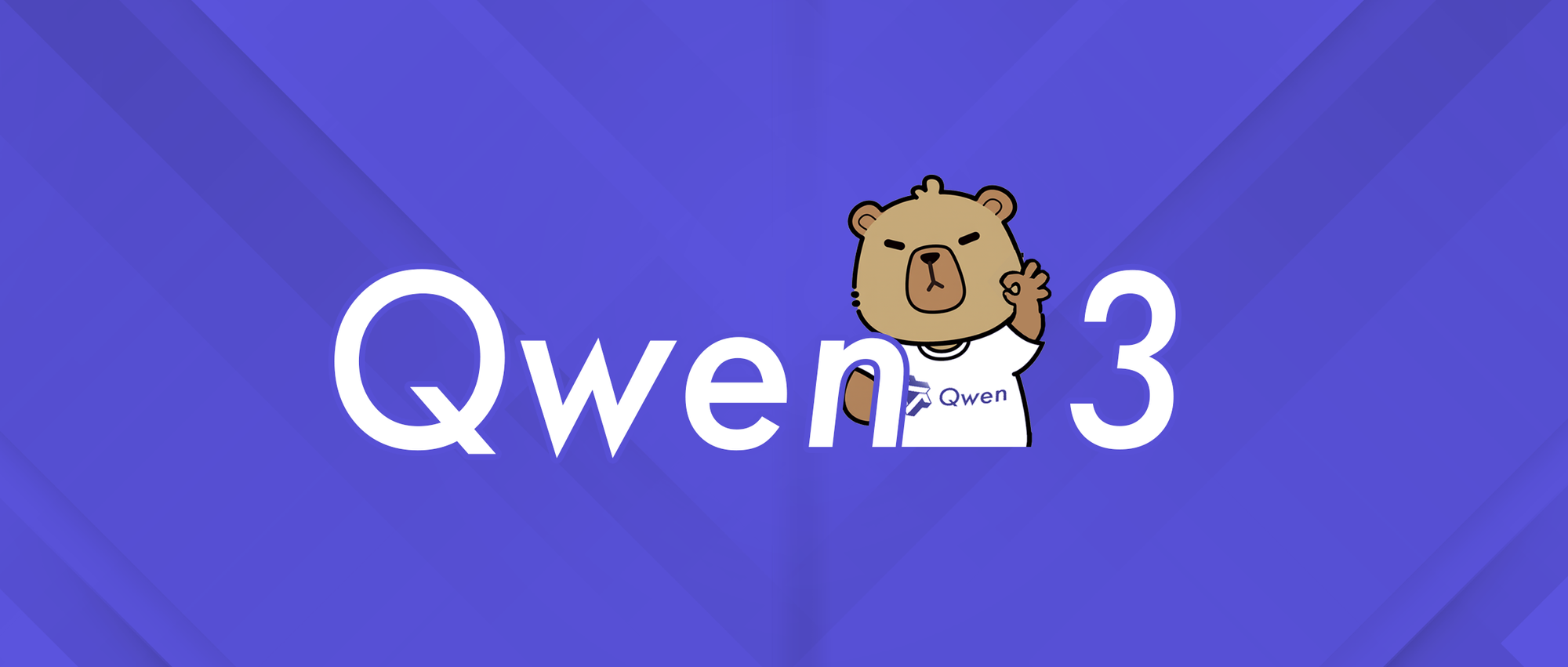
Qwen3-8B is one of the latest large language models (LLMs) from Alibaba's Qwen series, designed for high performance and versatility in a wide range of natural language processing tasks.
Running Qwen3-8B locally on Ubuntu allows developers and researchers to leverage its capabilities without relying on cloud APIs, ensuring data privacy, low latency, and cost efficiency.
What is Qwen3-8B?
Qwen3-8B is an 8-billion parameter transformer model, offering a balance between computational requirements and language understanding. It is available in various formats and can be deployed using multiple frameworks, such as Ollama, vLLM, Hugging Face Transformers, and more.
System Requirements
Before installing and running Qwen3-8B, ensure your system meets the following requirements:
- Operating System: Ubuntu 20.04 or newer (other Linux distros are also supported)
- CPU: x86_64 architecture
- RAM: At least 16 GB (32 GB recommended for smoother performance)
- GPU: NVIDIA GPU with at least 16 GB VRAM (e.g., RTX 3090, A6000, or better) for optimal performance; CPU-only inference is possible but significantly slower
- Storage: 20 GB free disk space (for model weights and dependencies)
- Internet Connection: Required for initial model download
Installation Methods
There are several ways to run Qwen3-8B on Ubuntu. The most popular and user-friendly methods are:
- Ollama: Easiest for beginners, abstracts most complexity1.
- vLLM: High-performance, optimized for serving LLMs at scale13.
- Hugging Face Transformers: Flexible for custom pipelines and research23.
- llama.cpp / llama-cpp-python: Lightweight, supports quantized models for CPU/GPU.
Running Qwen3-8B with Ollama
Ollama is a streamlined tool for running and managing LLMs locally. It handles model downloads, updates, and provides a simple CLI and API server.
Installation Steps
Install Ollama
- Visit the official Ollama website and follow the Linux installation instructions1.
- For Ubuntu, you can usually install with:bash
curl -fsSL https://ollama.com/install.sh | sh
Pull the Qwen3-8B Model
- Use the command:bashollama run qwen3:8b
- If the model isn't present locally, Ollama will automatically download it.
Run the Model
- After downloading, Ollama will start an interactive chat session.
- You can also use the API endpoint Ollama provides for programmatic access.
Advantages of Using Ollama
- Simplicity: One command to run the model.
- Automatic Updates: Keeps models and dependencies up to date.
- API Access: Easily integrate with applications.
Running Qwen3-8B with vLLM
vLLM is a high-throughput inference engine designed for serving LLMs efficiently, supporting advanced features like tensor parallelism and quantization13.
Installation Steps
- Install vLLM
- Ensure you have Python 3.8+ and pip installed.
- (Optional) Create a virtual environment:bashpython3 -m venv qwen3_env
source
qwen3_env/bin/activate - Install vLLM:bash
pip install
-U vllm - Install CUDA toolkit and drivers if using GPU2.
- Download and Serve Qwen3-8B
- Run the following command to start an OpenAI-compatible API server:bashvllm serve Qwen/Qwen3-8B --enable-reasoning --reasoning-parser deepseek_r1
- This will automatically download the model from Hugging Face if not present locally.
- Run the following command to start an OpenAI-compatible API server:bashvllm serve Qwen/Qwen3-8B --enable-reasoning --reasoning-parser deepseek_r1
- Access the Model
- The server will be available at
http://localhost:8000/v1/
. - You can interact with it using OpenAI-compatible clients or
curl
.
- The server will be available at
Advanced vLLM Options
- Tensor Parallelism: Use multiple GPUs for faster inference.
- Quantization: Reduce memory usage with FP8 or INT4 quantization.
- Custom Context Length: Adjust
--max-model-len
for longer prompts5.
Example API Call
bashcurl -X POST http://localhost:8000/v1/chat/completions \
'{
-H "Content-Type: application/json" \
-d
"model": "Qwen/Qwen3-8B",
"messages": [{"role": "user", "content": "Hello, Qwen3-8B!"}]}'
Running Qwen3-8B with Hugging Face Transformers
For those who want more control or wish to fine-tune the model, Hugging Face Transformers is the go-to library.
Installation Steps
- Install Dependenciesbash
sudo apt update && sudo apt
upgrade -ysudo apt install -y python3 python3-pip git
torch torchvision torchaudio --index-url https://download.pytorch.org/whl/cu118
pip installpip install
transformers - Set Up a Virtual Environment (Recommended)bashpython3 -m venv qwen_env
source
qwen_env/bin/activate - Download and Run Qwen3-8Bpython
from transformers import AutoModelForCausalLM,
AutoTokenizermodel_name = "Qwen/Qwen3-8B"
tokenizer = AutoTokenizer.from_pretrained(model_name)
model = AutoModelForCausalLM.from_pretrained(model_name, torch_dtype="auto", device_map="auto")
prompt = "What are the main features of Qwen3-8B?"
inputs = tokenizer(prompt, return_tensors="pt").to(model.device)
outputs = model.generate(**inputs, max_new_tokens=100)
print(tokenizer.decode(outputs[0], skip_special_tokens=True)) - (Optional) Use Quantized Models
- For systems with limited VRAM, use quantized versions (e.g., 4-bit, 8-bit) via
bitsandbytes
or GGUF format.
- For systems with limited VRAM, use quantized versions (e.g., 4-bit, 8-bit) via
Running Qwen3-8B with llama.cpp / llama-cpp-python
For lightweight inference, especially on CPUs or with quantized models, llama.cpp is a popular choice.
Installation Steps
- Install llama.cppbash
git
clone https://github.com/ggerganov/llama.cppcd
llama.cppmake
- Download GGUF Quantized Qwen3-8B Model
- Obtain the GGUF quantized model from Hugging Face or a trusted source.
- Run the Modelbash
./main -m qwen3-8b.gguf -p "Hello, Qwen3-8B!"
- Adjust parameters for context length, thread count, etc.
Performance Optimization Tips
- Use Quantized Models: Reduces VRAM and RAM requirements, often with minimal loss in quality5.
- Leverage GPU Acceleration: For best performance, use a modern NVIDIA GPU with sufficient VRAM.
- Adjust Batch Size and Context Length: Tweak these parameters based on your hardware to avoid out-of-memory errors56.
- Monitor Resource Usage: Use
nvidia-smi
andhtop
to monitor GPU and CPU usage.
Troubleshooting Common Issues
Out of Memory (OOM) Errors:
- Lower the batch size or context length.
- Use a more aggressively quantized model (e.g., 4-bit).
- Ensure swap space is enabled on your system.
Slow Inference:
Model Not Downloading:
- Check your internet connection and available disk space.
- Ensure you have the correct permissions to write to the model cache directory.
Comparing Deployment Methods
Method | Ease of Use | Performance | Flexibility | Best For |
---|---|---|---|---|
Ollama | ★★★★★ | ★★★★☆ | ★★★☆☆ | Beginners, quick setup |
vLLM | ★★★★☆ | ★★★★★ | ★★★★☆ | Production, high throughput |
Transformers | ★★★☆☆ | ★★★☆☆ | ★★★★★ | Research, customization |
llama.cpp | ★★★★☆ | ★★★☆☆ | ★★★☆☆ | Lightweight, quantized |
Advanced Topics
Fine-tuning Qwen3-8B
- While Qwen3-8B can be fine-tuned for specific tasks, this requires significant computational resources and expertise.
- Use Hugging Face's
Trainer
class or frameworks like LoRA for parameter-efficient fine-tuning.
Serving Qwen3-8B as an API
- Both Ollama and vLLM provide OpenAI-compatible API endpoints, allowing easy integration with existing applications.
- You can also deploy behind a reverse proxy (e.g., Nginx) for production use.
Scaling Across Multiple GPUs
- vLLM supports tensor parallelism, enabling you to split the model across multiple GPUs for faster inference and larger context windows13.
Best Practices and Recommendations
- Start Small: Begin with the default settings and scale up as needed.
- Use Virtual Environments: Avoid dependency conflicts by isolating your Python environment.
- Keep Drivers Updated: Ensure your NVIDIA drivers and CUDA toolkit match the requirements of your chosen inference engine.
- Monitor Community Updates: The Qwen and LLM communities are rapidly evolving; stay up to date for new features and optimizations.
Conclusion
Running Qwen3-8B on Ubuntu is now accessible to anyone with a modern workstation or server. Whether you prefer the simplicity of Ollama, the speed of vLLM, or the flexibility of Hugging Face Transformers, you can deploy this powerful LLM for research, prototyping, or production workloads.