Running DeepSeek's Janus-Pro-7B Model on AWS: Step-by-Step Guide
Learn how to deploy DeepSeek's Janus-Pro-7B multimodal AI model on AWS with this step-by-step guide. Optimize performance, reduce costs, and integrate AWS services like EC2, S3, and SageMaker.
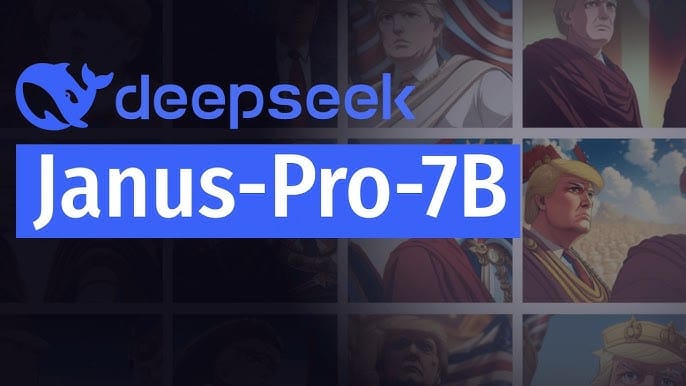
Running DeepSeek's Janus-Pro-7B model on Amazon Web Services (AWS) involves setting up the right environment, selecting the necessary AWS services, and deploying the model effectively.
This guide walks you through setup, optimization, and advanced use cases—perfect for developers and businesses seeking scalable AI solutions.
What is DeepSeek’s Janus-Pro-7B?
Janus-Pro-7B is a cutting-edge multimodal AI model that processes text and images for tasks like content generation, visual question answering, and more. Key advantages include:
- 🌟 Unified Architecture: Combines image/text processing in a single transformer model.
- ⚡ Cost Efficiency: 30% faster inference than Stable Diffusion XL, reducing cloud compute costs.
- 🖼️ High-Quality Output: Trained on 100M+ multimodal data points for superior results.
Why AWS for Janus-Pro-7B?
AWS provides scalable infrastructure tailored for AI/ML workloads:
- GPU-Powered Instances: Optimized for deep learning (e.g., p4d.24xlarge with 8x NVIDIA A100 GPUs).
- Managed Services: SageMaker simplifies model deployment.
- Global Availability: Deploy in 32 regions for low-latency applications.
Step 1: AWS Environment Setup
Choose Your Compute Service
Service | Best For | Estimated Cost (Hourly) |
---|---|---|
EC2 (g5.xlarge) | Customizable GPU workloads | $1.006 |
SageMaker | Managed ML pipelines | $1.20 (ml.g5.xlarge) |
Recommended: Start with EC2 for full control, then migrate to SageMaker for production.
Launch an EC2 Instance
- Select AMI: Use Deep Learning AMI (Ubuntu 20.04) for pre-installed ML tools.
- Choose an Instance Type – Opt for GPU-enabled instances like
p3
org4
for optimal performance. - Configure Instance Details – Assign IAM roles for S3 and other AWS services.
- Instance Type:
- For testing: g4dn.xlarge (1x T4 GPU, 4 vCPUs)
- Production: p3.8xlarge (4x V100 GPUs)
- Storage: Attach 100GB GP3 SSD for model weights.

Configure Security
- Create an IAM role with permissions for S3, CloudWatch, and SageMaker.
- Enable SSH access via key pairs.
- Set up a VPC with restricted inbound rules.
Step 2: Install Dependencies
Install Required Software
Once your EC2 instance is running:
Clone the Janus-Pro Repository:
git clone https://github.com/deepseek-ai/Janus.git
cd Janus
Install PyTorch and Transformers:
pip install torch torchvision torchaudio transformers
Install Python and Pip:
sudo apt update
sudo apt install python3 python3-pip
Connect via SSH:
ssh -i your-key.pem ec2-user@your-instance-ip
Download the Model
Retrieve Janus-Pro-7B from Hugging Face:
pip install huggingface_hub
from huggingface_hub import hf_hub_download
model_path = hf_hub_download("deepseek-ai/Janus-Pro-7B")
Connect via SSH and run:
# Update packages
sudo apt update && sudo apt upgrade -y
# Install Python 3.10
sudo apt install python3.10 python3-pip -y
# Set up virtual environment
python3 -m venv janus-env
source janus-env/bin/activate
# Install PyTorch with CUDA 11.7
pip3 install torch torchvision torchaudio --extra-index-url https://download.pytorch.org/whl/cu117
# Clone Janus-Pro repo
git clone https://github.com/deepseek-ai/Janus.git
cd Janus && pip install -r requirements.txt
Step 3: Deploy the Model
Option A: Direct Download from Hugging Face
from huggingface_hub import snapshot_download
snapshot_download(
"deepseek-ai/Janus-Pro-7B",
local_dir="/models/janus-pro-7b",
token="YOUR_HF_TOKEN" # Get from Hugging Face settings
)
Option B: S3 Model Storage
- Create an S3 Bucket via the AWS Management Console.
- Upload Data – Store datasets needed for inference.
- Set Permissions – Attach an IAM role that allows your EC2 instance to access S3.
Mount S3 to EC2 using s3fs:
sudo apt install s3fs
echo ACCESS_KEY:SECRET_KEY > ~/.passwd-s3fs
chmod 600 ~/.passwd-s3fs
s3fs your-bucket /mnt/janus-model -o passwd_file=~/.passwd-s3fs
Upload model to S3:
aws s3 sync /models/janus-pro-7b s3://your-bucket/janus-pro-7b
Step 4: Run Inference
Basic Text-to-Image Generation
from janus import JanusPipeline
pipe = JanusPipeline.from_pretrained("/mnt/janus-model")
image = pipe("A futuristic city at sunset").images[0]
image.save("output.jpg")
Multimodal Input Example
from PIL import Image
prompt = """
USER: What's in this image?
ASSISTANT: <image>
"""
input_image = Image.open("street.jpg")
result = pipe(prompt, images=[input_image], max_new_tokens=200)
print(result.text) # Output: "The image shows a busy city street with..."
Advanced AWS Integrations
1. Auto-Scaling with SageMaker
Create an endpoint that scales based on demand:
from sagemaker.huggingface import HuggingFaceModel
model = HuggingFaceModel(
role='sagemaker-role',
transformers_version='4.28',
pytorch_version='2.0',
model_data='s3://your-bucket/janus-pro-7b.tar.gz',
)
predictor = model.deploy(
initial_instance_count=1,
instance_type='ml.g5.12xlarge'
)
2. Serverless API with Lambda
Trigger model inference via API Gateway:
import json
import boto3
def lambda_handler(event, context):
sagemaker = boto3.client('sagemaker-runtime')
response = sagemaker.invoke_endpoint(
EndpointName='janus-pro-endpoint',
Body=json.dumps(event['body']),
ContentType='application/json'
)
return {
'statusCode': 200,
'body': response['Body'].read().decode()
}
Setting Up AWS for Janus-Pro-7B
Choosing the Right AWS Services
To efficiently run Janus-Pro-7B, you need the following AWS services:
- Amazon EC2 (Elastic Compute Cloud) – Provides scalable computing capacity.
- Amazon S3 (Simple Storage Service) – Stores model artifacts and datasets.
- Amazon EFS (Elastic File System) – Offers shared storage across multiple instances.
- AWS Lambda – Enables serverless execution of small tasks.
- Amazon SageMaker – Facilitates model training and deployment.
Running Inference with Janus-Pro-7B
Example Code for Text Inference
import torch
from transformers import AutoModelForCausalLM, AutoTokenizer
# Load the model and tokenizer
model = AutoModelForCausalLM.from_pretrained("deepseek-ai/Janus-Pro-7B")
tokenizer = AutoTokenizer.from_pretrained("deepseek-ai/Janus-Pro-7B")
# Prepare input text
input_text = "Describe what you want the image to depict."
inputs = tokenizer(input_text, return_tensors="pt")
# Generate output
with torch.no_grad():
outputs = model.generate(**inputs)
# Decode and print output
result = tokenizer.decode(outputs[0], skip_special_tokens=True)
print(result)
Handling Image Inputs
For image processing, additional libraries like PIL or OpenCV are required:
from PIL import Image
# Load an image
image = Image.open("path_to_your_image.jpg")
Advanced Usage Scenarios
Batch Processing
For processing multiple text inputs simultaneously:
batch_inputs = [tokenizer("Input text 1", return_tensors="pt"),
tokenizer("Input text 2", return_tensors="pt")]
# Process batch inputs
outputs = [model.generate(**input) for input in batch_inputs]
Integrating with AWS Services
- AWS Lambda – Trigger functions when new data is uploaded to S3.
- Amazon API Gateway – Expose the model as an API for external applications.
Monitoring and Scaling Your Application
- CloudWatch Dashboard:
- Track GPU utilization (%)
- Monitor
ModelLatency
metric (keep <500ms)
- Common Errors:
- CUDA Out of Memory: Reduce batch size or upgrade to p4d instances.
- Model Loading Failures: Verify S3 bucket permissions with IAM.
- Autoscaling EC2 Instances
- For dynamic workloads:
- Set up an Auto Scaling group.
- Define scaling policies based on CloudWatch metrics.
Cost Optimization Tips
- Spot Instances: Save 70% on EC2 costs for batch jobs.
- S3 Intelligent Tiering: Automatically moves unused model weights to cheaper storage.
- Inference Recommender: Use SageMaker’s tool to find the most cost-effective instance type.
Conclusion
Deploying DeepSeek’s Janus-Pro-7B on AWS enables robust multimodal AI applications. By leveraging AWS services effectively, you can scale efficiently while optimizing costs. This guide provides a comprehensive roadmap to setting up, deploying, and managing Janus-Pro-7B on AWS for successful AI-powered implementations.