Running Kimi-Audio on Mac: A Complete Guide
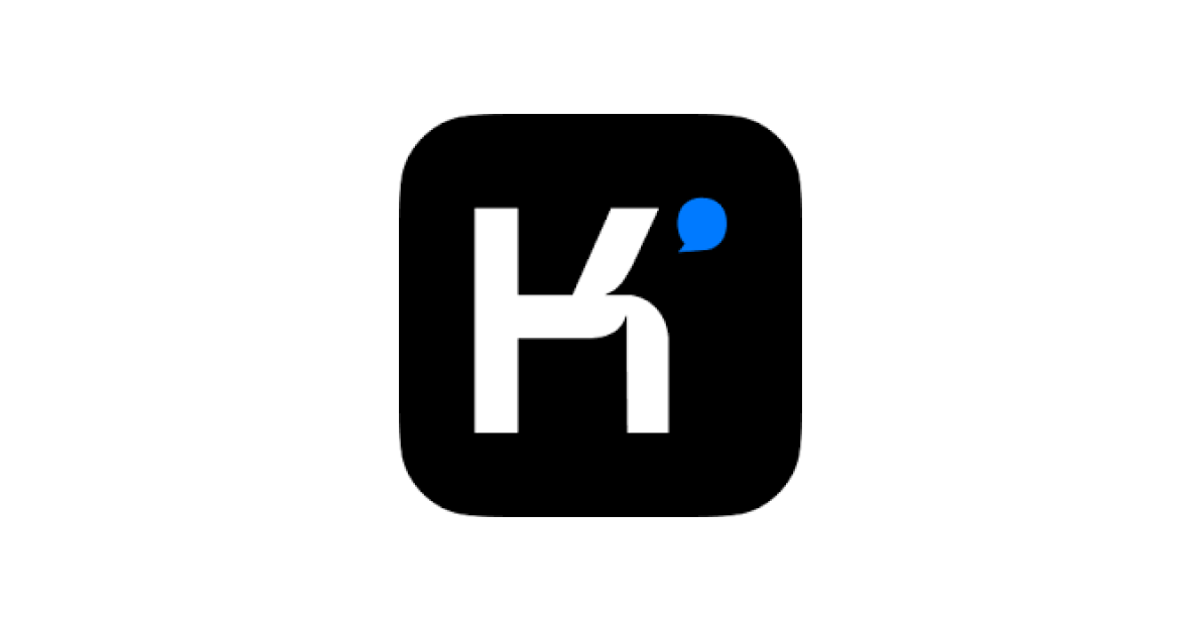
Kimi-Audio is a universal audio foundation model capable of audio understanding, generation, and processing. Kimi-Audio is an open-source AI model designed for audio-to-text (ASR) and audio-to-audio/text conversation tasks
This guide adapts the workflow for macOSSystem Requirements and Compatibility
Hardware Requirements
- Apple Silicon (M1/M2/M3) or Intel-based Mac:
- M-series chips are preferred for optimized performance.
- Intel Macs require Rosetta 2 for x86 compatibility.
- RAM: Minimum 16GB (32GB recommended for large models).
- Storage: 20GB free space for models and dependencies.
Software Requirements
- macOS 12.0 or later (Ventura, Sonoma, or Sequoia).
- Python 3.8+ and
pip
for dependency management. - CUDA Support: Not natively available on macOS; use Core ML or CPU-based inference.
Installation Steps
Step 1: Set Up Developer Tools
- Install Xcode Command Line Tools:bashxcode-select --install
- Install Homebrew:bash
/bin/bash -c "$(curl -fsSL https://raw.githubusercontent.com/Homebrew/install/HEAD/install.sh)"
Step 2: Clone Kimi-Audio Repository
bashgit clone https://github.com/[Kimi-Audio-Repo].git # Replace with actual repo URL[^1]
Kimi-Audio
cd
Step 3: Create a Virtual Environment
bashpython3 -m venv kimi-envsource
kimi-env/bin/activate
Step 4: Install Dependencies
bashpip install -r requirements.txt # Adapt requirements for macOS compatibility[^1][4]
Alternate Method
A. You can load the Kimi-Audio model using the KimiAudio
class from the kimia_infer.api.kimia
module. Ensure you have the model path or the model ID from the Hugging Face Hub.
B. Define the sampling parameters for audio and text generation.
- Common Adjustments:
- Replace
torch
with Apple'storch-mps
for Metal Performance Shaders1:bashpip install
torch torchaudio - Use
onnxruntime
for CPU/GPU inference on Apple Silicon.
- Replace
Define Sampling Parameters:PythonCopy
sampling_params = {
"audio_temperature": 0.8,
"audio_top_k": 10,
"text_temperature": 0.0,
"text_top_k": 5,
"audio_repetition_penalty": 1.0,
"audio_repetition_window_size": 64,
"text_repetition_penalty": 1.0,
"text_repetition_window_size": 16,
}
Load the Model:PythonCopy
from kimia_infer.api.kimia import KimiAudio
model_path = "moonshotai/Kimi-Audio-7B-Instruct"
model = KimiAudio(model_path=model_path, load_detokenizer=True)
Live Examples
Example 1: Audio-to-Text (ASR)
- Prepare the Audio File:
- Ensure you have an audio file ready for transcription. For example,
asr_example.wav
.
- Ensure you have an audio file ready for transcription. For example,
Transcribe the Audio:PythonCopy
import soundfile as sf
messages_asr = [
{"role": "user", "message_type": "text", "content": "Please transcribe the following audio:"},
{"role": "user", "message_type": "audio", "content": "test_audios/asr_example.wav"}
]
_, text_output = model.generate(messages_asr, **sampling_params, output_type="text")
print(">>> ASR Output Text: ", text_output) # Expected output: "This is not a farewell, this is the end of one chapter and the beginning of a new one。" [^224^]
Example 2: Audio-to-Audio/Text Conversation
- Prepare the Audio File:
- Ensure you have an audio file ready for the conversation. For example,
qa_example.wav
.
- Ensure you have an audio file ready for the conversation. For example,
Generate Audio and Text Output:PythonCopy
messages_conversation = [
{"role": "user", "message_type": "audio", "content": "test_audios/qa_example.wav"}
]
wav_output, text_output = model.generate(messages_conversation, **sampling_params, output_type="both")
output_audio_path = "output_audio.wav"
sf.write(output_audio_path, wav_output.detach().cpu().view(-1).numpy(), 24000) # Assuming 24kHz output
print(f">>> Conversational Output Audio saved to: {output_audio_path}")
print(">>> Conversational Output Text: ", text_output) # Expected output: "A." [^224^]
Audio Device Configuration
CoreAudio Setup
- Configure Input/Output Devices:
- Navigate to System Settings > Sound to select devices.
- Use Audio MIDI Setup for advanced routing.
- Sample Rate Synchronization:
- Match sample rates (e.g., 44.1kHz or 48kHz) between Kimi-Audio and macOS.
Aggregate Devices for Multi-Channel I/O
- Open Audio MIDI Setup > Create Aggregate Device.
- Combine physical interfaces (e.g., Scarlett 18i20) with built-in microphones.
Running Kimi-Audio
Command-Line Execution
bashpython kimi_audio.py --input "What is happiness?"
--output_format both
- Flags:
--device mps
for Metal acceleration on Apple Silicon1.--precision fp16
to reduce memory usage1.
GUI Workflow (Hypothetical)
If a GUI is available:
- Launch the app and grant microphone access via System Settings > Privacy & Security7.
- Select input/output devices from dropdown menus.
Performance Optimization
Metal Performance Shaders (MPS)
- Enable MPS Backend:python
import
torchdevice = torch.device("mps")
- Monitor GPU Usage:
Use Activity Monitor > GPU History1.
Memory Management
- Reduce Batch Size: Lower
--batch_size
to 1-2 for long-form audio1. - Use
swapoff
: Disable swap to prevent SSD wear (only for 32GB+ RAM systems).
Troubleshooting
Common Issues
- Crackling Audio:
- Adjust sample rate in Audio MIDI Setup.
- Disconnect external devices.
- Installation Failures:
- Reinstall Xcode tools:
xcode-select --reset
. - Use Rosetta 2 terminal for Intel-specific dependencies.
- Reinstall Xcode tools:
Debugging Tools
- Console Logs: Filter for
coreaudiod
errors. - Test MIDI/Audio Routing: Use Audio MIDI Setup > Test MIDI6.
Advanced Use Cases
Fine-Tuning on macOS
- Convert datasets to Core ML format using
coremltools
. - Use
mlcompute
framework for on-device training.
API Integration
pythonfrom kimi_audio import
KimiModelmodel = KimiModel(device="mps")
model.generate("Explain quantum computing in 200 words.")
Alternatives and Complements
- Camel AI: For multi-agent text generation alongside Kimi-Audio1.
- Logic Pro Integration: Route Kimi’s output to DAWs via virtual audio cables.
Future-Proofing for macOS Sequoia
- Check Compatibility: Verify dependencies with Sweetwater’s macOS Sequoia Guide.
- Test Beta Builds: Use Apple’s TestFlight for early M3 optimizations.
Security Considerations
- Sandboxing: Run Kimi in a Docker container via OrbStack (Apple Silicon-native).
- Microphone Permissions: Audit via System Settings > Privacy.
Conclusion
Kimi-Audio provides a powerful and flexible solution for audio-to-text and audio-to-audio/text conversation tasks. By following the steps outlined above, you can easily set up and run Kimi-Audio on your Mac.
The model's capabilities make it suitable for a wide range of applications, from transcription services to interactive voice assistants. For more detailed information and additional examples, refer to the Kimi-Audio GitHub repository.